RDM
(→Big idea) |
(→Big idea) |
||
Line 9: | Line 9: | ||
=== RDM in general === | === RDM in general === | ||
− | Decision-making under uncertainty is not a new problem, but the way to approach and handle these often complex issues has changed over time. RDM has become one of the more common approaches to handle decision making under deep uncertainty. The first person to describe the RDM methodology was Robert J. Lempert in 1997 in "When we don't know the costs or the benefits: Adaptive strategies for abating climate change "<ref name="Lempert1997"> Lempert R.J., Schlesinger M.E., & Bankes S.C. (1996). When we don’t know the costs or the benefits: Adaptive strategies for abating climate change. Climatic Change, Volume 37, Issue 1, 207–208. </ref>. According to R.J Lempert, the traditional “predict-then-act” framework has proven to be successful for decades, which both a large number of theories and mathematical techniques is proof on. RDM was original developed to make policymakers able to make better decisions about issues that could have consequences in the long-term. RDM were more specifically focused on decision processes about climate changes and how this complex and very unpredictable problem could be handled, the reason for this is that climate change is commonly known as an issue embraced with deep uncertainty<ref =name"DMDU"> Marchau V.A.W.J., Walker W.E., Bloemen P.J.T.M, Popper S.W (2019), Decision Making under Deep Uncertainty, From Theory to Practice, Springer Cham, DOI: https://doi.org/10.1007/978-3-030-05252-2</ref>. RDM were therefore a particularly useful alternative for finding suitable strategies regarding to the area of climate changes. . The need for RDM is therefore targeted more towards complex decision processes under deep uncertainty, where relatively simple prediction analysis is not enough and often will be misleading. R.J. Lempert thought it would be better to seek a robust solution rather than trying to predict the future and then choose the solution that fits the predicted future best. He disagreed with the traditional framework for assessing alternatives in decision processes, in which he thinks predictions were imposed too great a significance. He argued that the reason for decision-makers to use predictions were to make the future less scary and that the ''"the quest for prediction probably fills some deep human need. Even though the accuracy of most predictions has proven to be poor"''"<ref name="Lempert2000">Lempert R.J., Schlesinger M.E. (2000) Robust strategies for abating climate change. Climatic Change, Springer Netherlands, Volume 45, Page 387–401, DOI: 10.1023/a:1005698407365 </ref>. A combination of new technologies and the need for a method to handle more complex issues resulted in the development of RDM. Instead of finding the alternative that seems to be the best based on specific predictions, decision-makers can now use modern technology to analyse and evaluate alternatives against thousands or even millions of possible scenarios. The RDM method gives the decision-maker a tool to identify trade-offs when testing strategies against different scenarios. In that way, decision-makers can now determine which strategies that perform best under a large range of possible scenarios. It results in not necessarily the best strategy, but the most robust strategy that can handle the future, regardless of how the future ends up being. | + | Decision-making under uncertainty is not a new problem, but the way to approach and handle these often complex issues has changed over time. RDM has become one of the more common approaches to handle decision making under deep uncertainty. The first person to describe the RDM methodology was Robert J. Lempert in 1997 in "When we don't know the costs or the benefits: Adaptive strategies for abating climate change "<ref name="Lempert1997"> Lempert R.J., Schlesinger M.E., & Bankes S.C. (1996). When we don’t know the costs or the benefits: Adaptive strategies for abating climate change. Climatic Change, Volume 37, Issue 1, 207–208. </ref>. According to R.J Lempert, the traditional “predict-then-act” framework has proven to be successful for decades, which both a large number of theories and mathematical techniques is proof on. RDM was original developed to make policymakers able to make better decisions about issues that could have consequences in the long-term. RDM were more specifically focused on decision processes about climate changes and how this complex and very unpredictable problem could be handled, the reason for this is that climate change is commonly known as an issue embraced with deep uncertainty<ref =name"DMDU"> Marchau V.A.W.J., Walker W.E., Bloemen P.J.T.M, Popper S.W (2019), Decision Making under Deep Uncertainty, From Theory to Practice, Springer Cham, DOI: https://doi.org/10.1007/978-3-030-05252-2</ref>. RDM were therefore a particularly useful alternative for finding suitable strategies regarding to the area of climate changes. With the arrival of the RDM, it was no longer necessary to determine the probabilities of the future consequences of climate changes, in order to make a strategy, which many also believe is something near impossible. The need for RDM is therefore targeted more towards complex decision processes under deep uncertainty, where relatively simple prediction analysis is not enough and often will be misleading. R.J. Lempert thought it would be better to seek a robust solution rather than trying to predict the future and then choose the solution that fits the predicted future best. He disagreed with the traditional framework for assessing alternatives in decision processes, in which he thinks predictions were imposed too great a significance. He argued that the reason for decision-makers to use predictions were to make the future less scary and that the ''"the quest for prediction probably fills some deep human need. Even though the accuracy of most predictions has proven to be poor"''"<ref name="Lempert2000">Lempert R.J., Schlesinger M.E. (2000) Robust strategies for abating climate change. Climatic Change, Springer Netherlands, Volume 45, Page 387–401, DOI: 10.1023/a:1005698407365 </ref>. A combination of new technologies and the need for a method to handle more complex issues resulted in the development of RDM. Instead of finding the alternative that seems to be the best based on specific predictions, decision-makers can now use modern technology to analyse and evaluate alternatives against thousands or even millions of possible scenarios. The RDM method gives the decision-maker a tool to identify trade-offs when testing strategies against different scenarios. In that way, decision-makers can now determine which strategies that perform best under a large range of possible scenarios. It results in not necessarily the best strategy, but the most robust strategy that can handle the future, regardless of how the future ends up being. |
Revision as of 13:38, 16 February 2021
Contents |
Abstract
Robust decision-making (RDM), is a relatively new and innovative methodology in the science of decision support. The RDM framework is a key tool in decision-making processes under deep uncertainty and provides decision-makers with a method to make a plan for the future without having to predict it. When decision-makers have to make long-term decisions they're often called upon to anticipate future needs, resources and circumstances. The problem is that decisions made on predictions are less reliable the farther the prediction reach forward in time, as time entails the prediction to become more vulnerable to uncertainty. This uncertainty could e.g. be unforeseen economic crashes terrorism attacks, political instability, climate change, chains of actions or reactions leading to more possible outcomes than a single prediction can handle. In order to handle a large amount of possible futures, RDM uses computer simulations and advanced modelling techniques to stress-test strategies not only against one predicted future but against thousands or millions of possible futures [1]. Thus the RDM framework is used for Decision Making under Deep Uncertainty (DMDU) [2]. The main purpose of RDM is therefore not to make a better prediction but through its concepts and processes to contribute with knowledge for the decision-maker to be able to design more robust strategies, that perform no matter what the future holds[3].
This paper will go in-depth with the concept of RDM, the theory behind RDM and it's purpose. Further in-depth guidance on how RDM is implemented and used, as well as an analysis of when RDM is applicable, will be presented. Finally, a critical reflection on RDM will seek to find and enlighten the limitations of RDM.
Big idea
Describe the tool, concept or theory and explain its purpose. The section should reflect the current state of the art on the topic
RDM in general
Decision-making under uncertainty is not a new problem, but the way to approach and handle these often complex issues has changed over time. RDM has become one of the more common approaches to handle decision making under deep uncertainty. The first person to describe the RDM methodology was Robert J. Lempert in 1997 in "When we don't know the costs or the benefits: Adaptive strategies for abating climate change "[4]. According to R.J Lempert, the traditional “predict-then-act” framework has proven to be successful for decades, which both a large number of theories and mathematical techniques is proof on. RDM was original developed to make policymakers able to make better decisions about issues that could have consequences in the long-term. RDM were more specifically focused on decision processes about climate changes and how this complex and very unpredictable problem could be handled, the reason for this is that climate change is commonly known as an issue embraced with deep uncertainty[5]. RDM were therefore a particularly useful alternative for finding suitable strategies regarding to the area of climate changes. With the arrival of the RDM, it was no longer necessary to determine the probabilities of the future consequences of climate changes, in order to make a strategy, which many also believe is something near impossible. The need for RDM is therefore targeted more towards complex decision processes under deep uncertainty, where relatively simple prediction analysis is not enough and often will be misleading. R.J. Lempert thought it would be better to seek a robust solution rather than trying to predict the future and then choose the solution that fits the predicted future best. He disagreed with the traditional framework for assessing alternatives in decision processes, in which he thinks predictions were imposed too great a significance. He argued that the reason for decision-makers to use predictions were to make the future less scary and that the "the quest for prediction probably fills some deep human need. Even though the accuracy of most predictions has proven to be poor""[6]. A combination of new technologies and the need for a method to handle more complex issues resulted in the development of RDM. Instead of finding the alternative that seems to be the best based on specific predictions, decision-makers can now use modern technology to analyse and evaluate alternatives against thousands or even millions of possible scenarios. The RDM method gives the decision-maker a tool to identify trade-offs when testing strategies against different scenarios. In that way, decision-makers can now determine which strategies that perform best under a large range of possible scenarios. It results in not necessarily the best strategy, but the most robust strategy that can handle the future, regardless of how the future ends up being.
RDM consist of the following key elements:
- Multiple plausible futures. The combination of scenarios for the future should be very diverse. The reason for this is that the main idea of RDM is that you are interested in analysing how different strategies perform in as many different futures as possible. These multiple scenarios can also correspond to different person view of the world.
- The goal is not to find the best strategies but to find the most robust ones. The main focus in RDM is to find strategies that perform well over most if not all possible scenarios.
- Apply adaptive strategies. One way to secure that strategies are robust is to choose some who adaptive. Adaptive means that when the future comes, the strategies can adapt to the new circumstances, and still perform well under the new conditions.
- The computer is used to ease the human job, not to give the final recommendation of which specific strategy that should be used.
Theory
Decisionmakers, and the analysts upon whom they rely, have had good reason to feel decreasing confidence in their ability to anticipate correctly future technological, economic, and social developments, future changes in the system they are trying to improve, or the multiplicity and time-varying preferences of stakeholders regarding the system’s outcomes.
Consider, for example, decisionmaking related to the conse- quences of climate change, the future demand for and means for providing mobility, the planning of mega-scale infrastructure projects, the selection of energy sources to rely on in the future, the role of genomics in healthcare, or how cities will develop. Or think of rare events like a natural disaster, a financial crisis, or a terrorist attack. These topics are all characterized by what can be called “deep uncertainty.”
Application
Provide guidance on how to use the tool, concept or theory and when it is applicable
Steps in RDM
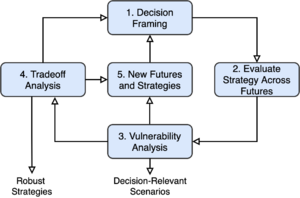
Step 1. Decision Framing The stakeholders start out with a decision framing exercise, in which they have to define the key factors relevant in the further analysis:
- Objectives and criteria
- Strategies/Alternatives that successfully can handle the objectives
- Uncertainties and their consequences
- Relationship between strategies, objectives and uncertainties
Step 2. Evaluate Strategy Across Futures
Next step is to use an "agree-on-decision" approach". To do so, RDM uses simulations of the strategies found in Step 1, against a variety of plausible scenarios of the future. These simulations generate a large number of results, which is merged into a large database[1].
Step 3 Vulnerability Analysis
Step 3 tries to identify, explore and characterize vulnerabilities. The decision-making team can use visualisations and data analytics based on the database made in step 2. In order to identify the key factors that contribute to the success or failure of the strategies, it is common practice to use Scenario Discovery (SD) algorithms. The use of SD algorithms will result in clusters of futures that will enlighten which factors the strategies are most vulnerable to[1].
Step 4 Tradeoff Analysis
A tradeoff analysis is performed on the scenarios from step 3. In step 4 different strategies are evaluated in order to analyse the tradeoffs among different strategies. This is often done by making multi-objective tradeoff plots in which tradeoff curves can be used to e.g. compare cost against reliability for different scenarios. The tradeoff curves can be used by the decision-makers to determine what balance they evaluate to be most suitable among the objectives defined in step 1[1].
Step 5 New Futures and Strategies
Finally, the decision-making team can then use the findings from step 4 to evaluate if they have found any robust strategies, with tradeoffs that are better than for the existing strategies. The final strategies from the RDM will often be what you call, Adaptive Decision Strategies, which are strategies that adapt depending on how the future develops. The adaptive strategies consist of a short-term strategy with actions to be taken in the near future and so-called signpost which are predesignated signals that when observed, certain actions will be taken. These adaptive strategies can either be established from a jury of experts[7], or the most suitable combination of near-term actions, signposts and actions to be taken if the signals are observed, can be determined with the help of optimization algorithms[8].
Bennefits of the RDM methodogy
Limitations
Critically reflect on the tool/concept/theory. When possible, substantiate your claims with literature
RDM is not an easy method to follow and it certainly has it's requirements in order to be performed correctly, but as the philosophy behind RDM is " that it is better to be roughly right than precisely wrong", the models used to perform the RDM is relatively simple and fast models[10].
One of the limitations of
RDM approaches adopt the philosophy that it is better to be roughly right than precisely wrong, by working with relatively fast and simple models or fit for purpose models (Haasnoot et al., 2014) and avoiding complex and detailed modelling processes (Walker et al., 2013). An example of such an approach is expert judgment which does not rely on intensive quantitative data analysis. However, for analysing potential consequences of a large number of scenarios, correspondingly large data requirements, computational capability, model simulation and visualization, becomes necessary
As RDM uses probabilistic modelling there has to be used computers and it requires some kind of quantitative information. Moreover, it is quite complicated to perform the modelling which means that you have to be an expert otherwise you will have to get help from an external expert to perform it. https://econadapt-toolbox.eu/robust-decision-making
Annotated bibliography
Provide key references (3-10), where a reader can find additional information on the subject.
Bibliography
- ↑ 1.0 1.1 1.2 1.3 1.4 Lempert R.J. (2019) Robust Decision Making (RDM). In: Marchau V., Walker W., Bloemen P., Popper S. (eds) Decision Making under Deep Uncertainty. Springer, Cham. https://doi.org/10.1007/978-3-030-05252-2_2.
- ↑ Walker W.E., Lempert R.J., Kwakkel J.H. (2013) Deep Uncertainty. In: Gass S.I., Fu M.C. (eds) Encyclopedia of Operations Research and Management Science. Springer, Boston, MA. https://doi.org/10.1007/978-1-4419-1153-7_1140
- ↑ Lempert R.J., Groves D.G. (2010) Identifying and evaluating robust adaptive policy responses to climate change for water management agencies in the American west, Technological Forecasting and Social Change, Volume 77, Issue 6, Pages 960-974, ISSN 0040-1625, https://doi.org/10.1016/j.techfore.2010.04.007.
- ↑ Lempert R.J., Schlesinger M.E., & Bankes S.C. (1996). When we don’t know the costs or the benefits: Adaptive strategies for abating climate change. Climatic Change, Volume 37, Issue 1, 207–208.
- ↑ Marchau V.A.W.J., Walker W.E., Bloemen P.J.T.M, Popper S.W (2019), Decision Making under Deep Uncertainty, From Theory to Practice, Springer Cham, DOI: https://doi.org/10.1007/978-3-030-05252-2
- ↑ Lempert R.J., Schlesinger M.E. (2000) Robust strategies for abating climate change. Climatic Change, Springer Netherlands, Volume 45, Page 387–401, DOI: 10.1023/a:1005698407365
- ↑ Lempert R.J., Popper S.W., Bankes S.C. (2003), Shaping the next one hundred years: New methods for quantitative, long-term policy analysis, Santa Monica, CA, The RAND Corporation, Technological Forecasting and Social Change — 2004, Volume 71, Issue 3, Page 305-307
- ↑ Lempert R.J., Collins M. (2007), Managing the risk of uncertain threshold responses: Comparison of robust, optimum, and precautionary approaches. Risk Analysis, Volume 27, Issue 4, Page 1009–1026
- ↑ Lempert R.J., Groves D.G., Popper S.W., Bankes S.S. (2016) A General, Analytic Method for Generating Robust Strategies and Narrative Scenarios, Volume 52, Issue 4, https://doi-org.proxy.findit.dtu.dk/10.1287/mnsc.1050.0472.
- ↑ Bhave A.G., Conway D., Dessai S., Stainforth D.A. (2016) Barriers and opportunities for robust decision making approaches to support climate change adaptation in the developing world, Climate Risk Management, Volume 14, Pages 1-10, ISSN 2212-0963,
https://findit.dtu.dk/en/catalog/2281533929 - 7.2.1 Robust Decision Making
https://www.tandfonline.com/doi/abs/10.1080/09544820010031580 - Robust decision-making for engineering design