Robust Decision Making (RDM)
Abstract: In some situations, decisions on projects or program management must been made under deep uncertainty [1]. In this case, the classic risk management tools are not well adapted. Robust Decision Making (RDM) is one of the solutions which can be used to correctly consider this high level of uncertainty. In contrary of the classic risk management tools, RDM helps decision makers to choose not the optimal solution, but the solution (of set of solutions) which is the less bad regarding the set of possible future[1]. After having presented the general idea and main principles of RDM, this article aims to give a guideline so that the readers can implement it to their project. This includes a step-by-step methodology and a very short presentation of the type of tools needed. The relevance and limitations of RDM are discussed at the end of this article. In this article if nothing is specified, the notions used are defined according to the PMI standards [2].
Presentation of the RDM
General Idea
As defined by Dr Alistair Hunt , Robust decision making is “ a methodology which aims to identify adaptation options or strategies which can perform well over a wider range of possible futures. “. [3]. The particularity of this method compared to the classic risk management tools, is that the goal is not to find the optimal solution to a problem but to create a robust solution that is satisfactory for a high number of potential futures [4].
In other terms, RDM doesn’t consist of trying to find the most plausible future and adapt your strategy to it. Instead, the gist of this method is to consider many potential future situations (see next parts for more details) and to assess strategies regarding all the different scenarios. Then the ones which are most robust are valorized compare to those which are optimal in one specific situation [1]. This method conducts to choose not best but the less bad strategy. The general foundations and a step by step approach are described later in this article.
When to use it ?
A situation of uncertainties and/or ambiguity implies to have a limited and unclear knowledge about future events [2]. When those uncertainties are deep, anticipate precisely the future become so hard that the classic tools of predictions or risk management cannot been use efficiently. Indeed, a high level of uncertainties implies that it is not possible to put reliable statistics or probabilities distributions on possible futures scenarios. And classic approaches need it according to [2].An alternative is then to consider as many possible futures as possible in the evaluation process in order to reduce the risk of their solutions to be totally not adapted to the actual future which will happen.
As we mentioned it before, one particularity of RDM is to consider a very large number of scenarios in the decision process. Therefore, it is specifically adapted to the context of deep uncertainties. A review of the state-of-the art uses of RDM can show us that almost all the current projects which use it are living in a context of such high level of uncertainties.
More precisely, these deep uncertainties can appears mainly in three situations [1] . First if the contextual uncertainties are deep. That is to say if the characteristics of the context in which will evolve the project, program or portefolio cannot be precisely defined. It is for example the case when the prediction of the future include climate modelization. Indeed, global warming will have impact that it is really difficult to precisely describe and anticipate [5] . Then uncertainties increase when “the set of policies has more rather than fewer degrees of freedom” [1]. It means that the more policies can change and influence your project, the more difficult it is to anticipate future situations. Finally, another source of uncertainties is the disagreement which can exists between different experts on the fields concerned by your projects. Indeed, if there is as strong disagreement, it is then impossible to set only one reliable hypothesis. Then many possible futures must be considered.
The field of application we can find are numerous. Here is a non-exhaustive list [6] :defense, higher education, insurance, science and technology planning, counter-terrorism…Within the state of the art, RDM is very often used when a project, a program or a portfolio has to include climate change in the decision [3] .
RDM fondations
As mentioned in literature [7] RDM don’t use computer models and data as a prediction tool to anticipate the best estimate future. Instead, RDM use the principle of exploratory modelling [8] . It means that a lot of possible future with many different paths are generated in order to stress proposed strategies. The goal is not to rank the plausible scenarios but to explore as many of them as possible. The confrontations of the pre-decided strategies to a large range of scenarios are then used to create a database of KPI and outcomes. In this data base two main types of data can be found.
The first is all the relevant data to assess if one strategy is adapted to one specific future regarding the achievement of pre-defined goals. The data use to say if a strategy is adapted or not to a situation really depends on the situation. It exists a large literature which describes how to choose KPI.Give example of data which can be relevant. RDM can use these results and methodologies to include them in the process.
The second type of data is a description of the path used to generate the range of possible futures. That is to say, all the characteristic of one path which are relevant to differentiate it from another one. Those date are mostly generated by coupling algorithms able to generate scenarios and software which can explore those scenarios [4] - see steps-by-steps section for more info.
With this database[1]data scientists can then use analytics to identify what are the common characteristics of the futures which make a strategy miss or reach its goals. They can also put the light on the strengths and weaknesses of the strategy regarding a set of different scenarios. These two first steps can be repeated for a large variety of input strategies and assumptions. Contrary to many classic risk assessments tools, the output of analytics in RDM process is not a predefined solutions but a set of key features which identify under which assumptions, which policies and which scenarios a strategy reach its goals.
When all those features are known, they can be transmitted to the decisions makers who will realize a tradeoff analyses in order to come up with a robust strategy. Strategies can then be modified of combine in order to find one which is robust. That is to say for [1]“ones that meet multiple objectives over many scenarios “. The all process described above can be reiterated multiples time to stress again the new strategies and to come up with an even more robust decision.
Collaboration Human/machine
One important point of RDM is that it relies on a collaboration human-machine [1]. What make the strength of this method is to combine the power of human decision making and the computational power of computers. Computers are used to generate and explore many different scenarios which are themselves used to stress strategies imagined by human. When it comes to deep uncertainties with a huge number of assumptions to explore, computational power is absolutely needed to cope with the massive among of data to proceed. On the other hand, the decision part and the creation of potential tradeoff then only relies on decision makers who can use the output of the previous computer-oriented steps as a support. This mix between computer science and human intelligence implies to use a set of different tools. As we can see it through different applications of RDM, the RDM method only define which general type of tool you need for each step. The exact tools to use are context dependent and must be defined for every new project. A very quick overview and guidance regarding the appropriate type of tools to use are detailed under the step-by-step section.
Step by Step methodology
The aim of this section is to give a detailed guideline for the reader to be able to apply RDM to a new project, program, or portfolio management situation. Each sub-section presents a step of the process and briefly discuss the useful tools for this section. The presentation order is chronological. The different steps are based on the methodology defined by Robert Lempert [7]
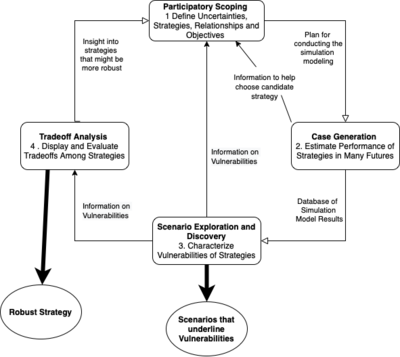
Define Strategies
As for every Projet, Program or Portefolio management situation, decision makers have to define a set of strategies. These strategies are probably going to evolve during the RDM process [9] but a foundation is needed to start the process. For a new project with high level of uncertainties, it may be useful to define a set of strategy that has to be tested instead of just one. Indeed, later the process will maybe lead to a combination of different strategies in order to get a robust one.
Define the uncertainties as much as possible
One of the crucial aspects to use correctly RDM, is to manage to be as comprehensive as possible in the description of the uncertainties. This is a huge foundation to unable simulation modeling to generate a lot of plausible solutions. The sources of uncertainties that must be explored are principally [1]:
- The contextual uncertainties. Basically, it is related to all the physical things included in your scope and for which you are not able to predict the evolution. One of the major examples of this part is all the strategies which must include climate or environmental simulations. Because it is not yet possible to have precise predictions, the level of uncertainties is very high.
- The policies uncertainties. This part includes all the things related to a decision making which can influence the result of your strategies and which are out of your control. For example, futures taxes, future environmental laws, future local restrictions etc… can be part of this category.
- The assumptions uncertainties. It may happen that experts disagree on some assumptions related to your strategies. If the disagreement is high, those assumptions generate uncertainties that you have to consider.
Set objectives and metrics for the Strategies
Later in the process, the goal will be to stress the different strategies with a large set of possible future and to evaluate if they are adapted or not. But a prerequire step is to define how this evaluations can be down [7]. At the end of this step, it is crucial to be clear on what have to be measured, how it has to down and what values defined an acceptable result.
Define a plan for conducting the simulation modeling
The simulation modeling is a key factor of RDM. Two things have to be down during the simulation [9]. First, the model has to be able to generate a large range of potential futures based on the uncertainties define previously. Second the model needs to give access to the different steps it is creating in all the potential futures. This is essential for enable humans to understand what are the impact of the hypothesis made at each steps on the strategies. The first point requires a scenario generator and the second an exploratory modeling software. It is then required to choose software adapted to the field and the scope of your project.
Also, your simulations will generate a lot of data. In order to make it usable for the next steps, it is crucial to define how this data will be collected and stored.
A last point which must be considered during the planning phase is the computational power requirement by the scenario generation. As mentioned in (find in one of the previous article the part on computational power) it can requires a lot of computational performances. And even if computers are making more and more progress, a too large number of scenarios may overtake the capacities you have or create a really long waiting time. Therefore, it is necessary to anticipate on those aspects and to reduce the complexity of the scenario generations if needed.
More information can be found on the references dedicated to exploratory modeling. [8]
Define a strategy of exploration and analyze of the data produced by the simulation modeling
When all the scenarios would have run, you will ended with a big database. And it is the exploration and the analyze of this database which will help to create value for RDM. So, it is important to have predefined strategies to exploit these data in an effective way. The method of RDM helps you to define what you are looking for. Indead [1] the goal is to identify and visualized the key factors of the scenarios which make your strategies work or not. In order to do this, classic tools of data analysis and machine learning can be used. You can also decide how you want to present your results. An important point to keep in mind is that you are not directly looking for one option to select. But you want to compare your different options. [1]
Run the simulation
This step only consists of applying the strategy defined in step D. The expected output is the data base mentioned previously and a way to visualize the path through the different futures.
Analyze and characterize the database produced
This step consists of applying the methodology defined in the point e. The expected outcome is an identification and a visualization of the key factors of the scenarios for which your strategies are work or not. The goal here is not to make a conclusion but to create a support which will help decision makers to do the tradeoff Analysis described below.
Realize a tradeoff Analysis
This step is where the value off the RDM in enlighten. During this phase, decision makers must use the results from the previous step to compare the different strategies. Specifically, the strengths and weaknesses of each method can be comparer considering the different scenarios. The goal is not to directly select a strategy. Instead, decision makers may want to see if it possible to combine two or more strategies in order to obtain a one which is more robust [1]. That is to say, a strategy which is better than the others when considering all the possible futures.
Iterate the process if necessary
After the tradeoff analysis, decision makers can come up with new strategies they would like to try or can review some assumptions used to creates the different scenarios. Then they can redo the all process and hope that it will help them to come up with a more robust solution. These new iterations are less time consuming because all the methodologies and the tools are the same than during the first one.
Limitations
As it a has been presented before, one of the particularities of RDM is to be well adapted to situations with a high level of uncertainties. But the relevance to use this method and not the classic probabilistic risk analysis is really limited when we are out of this context [7]. Indeed two mains points are often mentioned in the literature:
- RDM requires to have a high computational power because generating and exploring many possible futures have an high computational cost. [1]. So if the uncertainties are not deep and can be handle by the probabilistic approach, RDM will use unnecessary computational resources.
- The output of RDM is to provide a robust strategy. This strategy is then not necessary an optimal solution for one specific future but a tradeoff which minimize the negative aspects on many possible futures. In a context of lower uncertainties, i.e in a context where statistical tools can rank futures by probability, decision makers would prefer to find an optimal solution for the most probable futures. Then the idea of finding the less bad strategy become way less interesting than the idea of finding the best strategy. And then RDM is no more the method to prefer.
An other limitations to RDM is the complexity of the tools needed for creating and exploring the different scenarios [8] . First, those tools can be difficult to use and requires expert that should be part of the project. Second, it may appear that adapted scenarios generators don’t exist for very specific type of projects. In this case they must have to be implemented before to proceed to RDM. And this requires important human and financial resources.
Annotated Bibliography
Only the titles of the references are given in this section,. Links to articles can be found in the references section.
- Decision Making under Deep Uncertainty
This book offers a comprehensive examination of the approaches and tools for designing plans under deep uncertainty and their application. It identifies barriers and enablers for the use of the various approaches and tools in practice. More specifically, a section is dedicated to the analyse of Robust Decision Making.
References
- ↑ 1.00 1.01 1.02 1.03 1.04 1.05 1.06 1.07 1.08 1.09 1.10 1.11 1.12 Vincent A. W. J. MarchauWarren E. WalkerPieter J. T. M. BloemenSteven W. Popper (2019)Decision Making under Deep Uncertainty https://doi.org/10.1007/978-3-030-05252-2
- ↑ 2.0 2.1 2.2 Project Management: A guide to the Project Management Body of Knowledge (PMBOK guide), 7th Edition (2021)
- ↑ 3.0 3.1 Dr Alistair Hunt ROBUST DECISION MAKING https://econadapt-toolbox.eu/robust-decision-making
- ↑ 4.0 4.1 Jan H. Kwakkel, Marjolijn Haasnoot, Warren E. Walker,Comparing Robust Decision-Making and Dynamic Adaptive Policy Pathways for model-based decision support under deep uncertainty,Environmental Modelling & Software, Volume 86, 2016, Pages 168-183, ISSN 1364-8152, https://doi.org/10.1016/j.envsoft.2016.09.017
- ↑ Bhave, Ajay Gajanan, Conway, Declan, Dessai, Suraje and Stainforth, David A. (2016) Barriers and opportunities for robust decision making approaches to support climate change adaptation in the developing world. Climate Risk Management, 14 . pp. 1-10. ISSN 2212-0963 DOI: 10.1016/j.crm.2016.09.004
- ↑ Robert Lempert, Steven Popper, Steve Bankes. ROBUST DECISIONMAKING (RDM) https://millennium-project.org/wp-content/uploads/2020/02/22-Robust-Decisionmaking.pdf
- ↑ 7.0 7.1 7.2 7.3 7.4 Robert Lempert, Nidhi Kalra, Suzanne Peyraud, Zhimin Mao, Sinh Bach Tan, Dean Cira, Alexander Lotsch (2013) Ensuring Robust Flood Risk Management in Ho Chi Minh City https://openknowledge.worldbank.org/bitstream/handle/10986/15603/WPS6465.pdf?sequence=1&isAllowed=y
- ↑ 8.0 8.1 8.2 Agusdinata, Datu Buyung. (2008). Exploratory modeling and analysis: a promising method to deal with deep uncertainty.
- ↑ 9.0 9.1 Lempert, Robert J., Steven W. Popper, and Steven C. Bankes, Shaping the Next One Hundred Years: New Methods for Quantitative, Long-Term Policy Analysis, Santa Monica, Calif.: RAND Corporation, MR-1626-RPC, 2003. As of March 05, 2022: https://www.rand.org/pubs/monograph_reports/MR1626.html